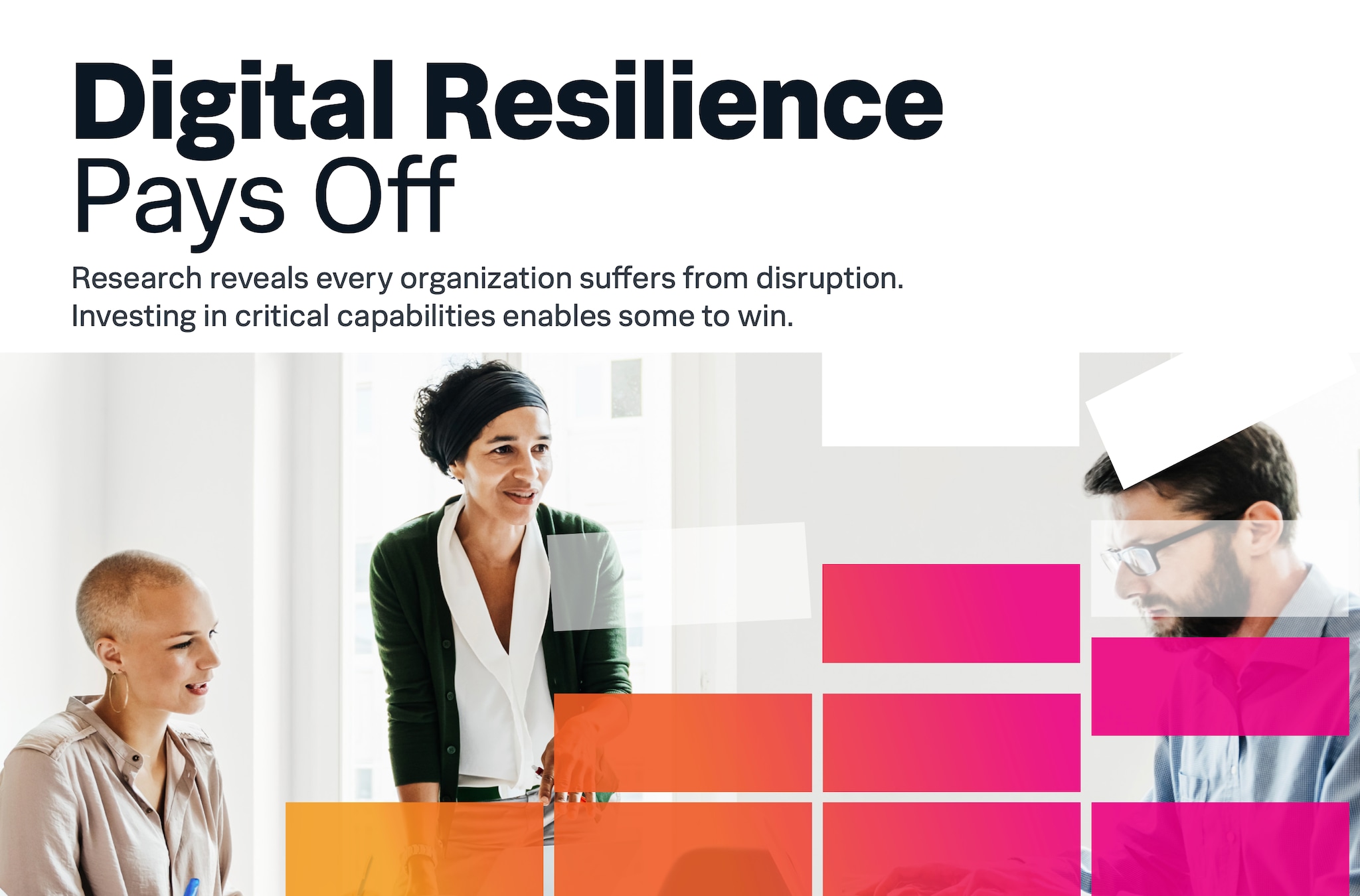
Digital Resilience Pays Off
Download this e-book to learn about the role of Digital Resilience across enterprises.
In the past few months, I have been asked a number of times whether we can determine what causes customers to feel that they have had a negative experience. This is an interesting question because a ‘negative customer experience’ can be defined in various ways, depending on the nature of the experience itself, and the data you have available to measure it. For example, it could be a low NPS score, a negative comment on social media, an escalation in the call center, or by a formal complaint.
What all of these examples have in common is that they are defined by the customer. The first step therefore in the process of understanding what drives negative customer experiences, is to establish a source of data that allows you to identify an appropriate definition. You also need to be able to correlate this information with other data sources which explains the reasons for this negativity.
To provide an example of how this analysis could be constructed, I am going to refer back to the SplunkTel upgrade customer journey demo (below) which I have discussed in previous blogs. This demo simulates the journey taken by customers who receive an offer to upgrade their mobile plan from their network operator in advance of their contract terminating.
In our demo, we used event gen to simulate data from the following sources:
By correlating these data sources, we have been able to generate a journey map showing how customers navigated their upgrade process – alongside the feedback they gave in a post-event survey.
In this example, we could define a negative customer experience as being where a customer gives a rating of 0-6 at the NPS survey question; the standard definition of a ‘detractor’. Based on this definition, we have created a series of dashboards, which show how we can start to build a picture of what causes detraction.
Dashboard 1 shows the number of detractors who completed the end-to-end upgrade customer journey in the last 24 hours.
The left shows the number of customers who completed a survey following their journey and were classified as detractors based on their feedback, as well as the change since yesterday. The right shows the total number of customers who completed this journey that our Machine Learning (logistic regression) model predicted as being detractors, based on their individual journey.
We used a RAG status (with static thresholds) to alert if either of these numbers should cause concern. In this case, there was a decline in both the number of actual and predicted detractors, so both KPIs are green: however, if there had been an increase, these numbers may have turned amber or red – depending on how our thresholds were set.
Dashboard 2 shows the daily count of the number of detractors over time, breaking this number down between actual detractors (taken from customer feedback) and predicted (taken from the ML model) on each day.
Dashboard 3 summarises the high level path that detractors took during their customer journey. In this output, our naming convention is based on what we feel was the defining phase of the journey.The most common paths are:
Dashboard 4 filters customers who have experienced an error. We have chosen to focus on those experiencing errors (even though this wasn’t the most common path) as this is arguably the group for which there is the greatest business imperative to follow up swiftly. This bar chart shows the value segment of those experiencing application or system errors.
Dashboard 5 shows a list of all ‘ultrahigh’ value segment customers who have experienced an error. For each customer, we have enriched the data with details of the offer that they received. This data could be sent directly to Customer Care teams for follow up, or alternatively to an EFM platform for further research, as appropriate.
This example shows how you can define a negative experience from the perspective of the consumer, as well as explore what is causing it, and who is affected. We also see how this information could be used to drive positive action (in this case a Customer Care call back or a follow up survey to understand more detail), with the aim of minimizing the business impact – and potentially even turning a negative experience into a positive one.
This has significant implications for delivering improved customer experience:
Thanks for reading!
Charles
The Splunk platform removes the barriers between data and action, empowering observability, IT and security teams to ensure their organizations are secure, resilient and innovative.
Founded in 2003, Splunk is a global company — with over 7,500 employees, Splunkers have received over 1,020 patents to date and availability in 21 regions around the world — and offers an open, extensible data platform that supports shared data across any environment so that all teams in an organization can get end-to-end visibility, with context, for every interaction and business process. Build a strong data foundation with Splunk.