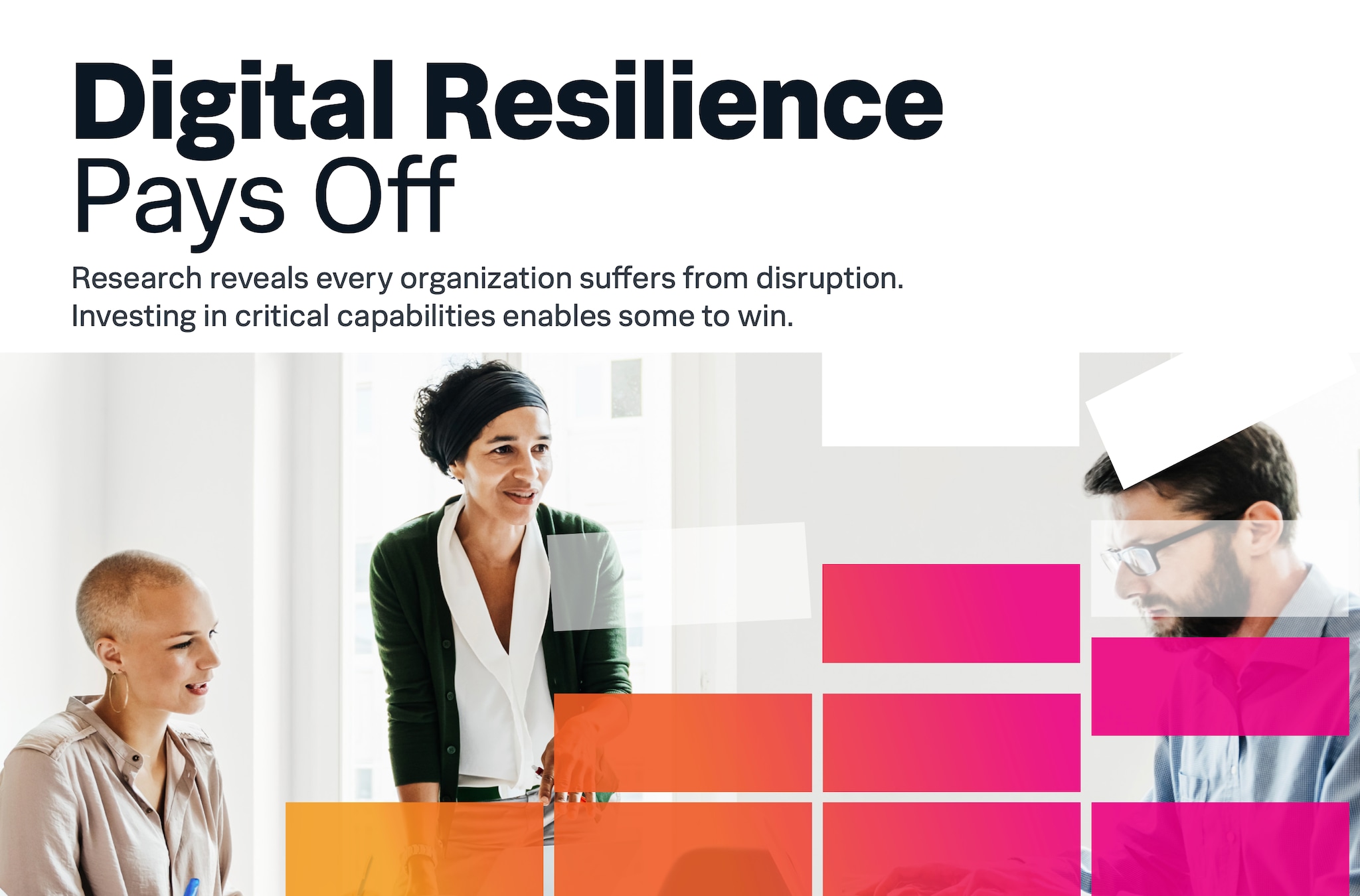
Digital Resilience Pays Off
Download this e-book to learn about the role of Digital Resilience across enterprises.
Historically, IT professionals have been sceptical of the role of machine learning with IT Operations. Many feel that the industry has been talking about it for almost 10 years, yet they still find themselves doing things manually, as they have always done.
In our everyday lives, we cannot pretend that machine learning doesn’t exist; whole business models are being based on it. For example; some retailers utilise it to proactively send customers clothing in the anticipation they will like and purchase items; it’s likely that you unlock your phone thanks to advancements in machine learning; and it even enables Google to sort through, tag, and classify your photos automatically, based on the objects detected (caravans, carnivals, cats…)
In terms of value, machine learning allows IT Operations to focus its time on the more enriching or higher-value tasks. Machine learning shouldn’t be different or difficult for these teams - in fact, I would say that it’s absolutely essential when faced with today’s IT challenges.
Applications are now loosely coupled with infrastructure; fragmented between physical, virtual and platform-as-a-service tiers, running within containers and based on microservices. Agile development, automation and infrastructure-as-code is accelerating this at ever increasing speeds. A modern application delivery toolchain is as complex and automated as a next-generation production line. Meanwhile, the reputation of a brand that in some cases has taken decades to build, can be damaged in moments, thanks to an outage or security vulnerability.
Closing the feedback loop with real-time insights is required at all stages of the application lifecycle to ensure continuous improvement. But as this is based on such large and complex data sets - how do humans keep up? The answer is that they simply can’t. Organisations must detect poor service quality immediately. Mean time to detect (MTTD) must be seconds, if not negative value - detecting an incident before it actually happens.
To gain the confidence of IT professionals, footsteps need to follow where organisations such as the likes of Google, have already been successful; developing packaged machine learning for very discrete, but high-value use cases. Event Management (EM) from the perspective of IT Operations would be a great example, for example. An efficient process of managing events is essential for any organisation that requires delivering top-quality services.
However, IT teams are struggling to manage the volume, complexity and unstructured nature of events generated by monitoring solutions. These events are essential to detect and triage incidents, but there are a number of challenges in managing, including:
Just like Google Photos, imagine if all your different alerts and events could be categorised and classified through unsupervised machine learning. Thresholds could be automatically set based on baselined behaviour and alerting instantly when there is an anomaly. This would mean less time spent managing the events, less events to analyse overall, and more time focused on what’s most important - improving your services.
Splunk is focused on packaging machine learning for discrete, high-value use cases within IT operations. Check out our whitepaper “Make Your IT Events Less Eventful” or try out the free ITSI Event Analytics sandbox.
----------------------------------------------------
Thanks!
Guillaume Ayme
The Splunk platform removes the barriers between data and action, empowering observability, IT and security teams to ensure their organizations are secure, resilient and innovative.
Founded in 2003, Splunk is a global company — with over 7,500 employees, Splunkers have received over 1,020 patents to date and availability in 21 regions around the world — and offers an open, extensible data platform that supports shared data across any environment so that all teams in an organization can get end-to-end visibility, with context, for every interaction and business process. Build a strong data foundation with Splunk.